Unraveling the Potential of AI in the Medical Practice How do we train the beast? An endless feed on data.
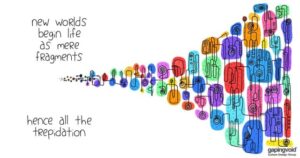
Data availability is a crucial factor in developing and deploying AI tools in healthcare, including those used in primary care. The potential of AI in healthcare relies heavily on access to high-quality, diverse, and well-annotated data. Here are some considerations regarding the availability of data for AI applications in primary care:
- Electronic Health Records (EHRs): EHR systems contain a wealth of patient data, including medical histories, diagnoses, treatments, and outcomes. Access to EHR and EMR data is valuable for building AI models for clinical decision support, risk prediction, and patient management. Currently, this data is largely locked away in 4000+ individual servers in back-room closets and under staircases in individual offices in this province. Less than 1/3 is in the cloud. Also, data is narrative-based, mostly unstructured, and rarely coded. Natural language processing and AI tools can make great sense of this text-based data and create information that can then be liberated and shared for use at the health system level, clinical decision support, and quality improvement.
- Medical Imaging Data: Hospitals and clinics generate vast amounts of medical imaging data, such as X-rays, MRIs, and CT scans. These images can be used for AI-driven diagnostic support and radiology image analysis. AI is already fantastic at “reading around the edges” of scans, finding things that human eyes miss as we zero in on the organs or pathology of interest. As data from images are amalgamated, this produces even better comparators for algorithms to find new diseases and anatomical variations. This makes it much easier for our tools to show only what is interesting and important in each individual patient. Machine learning from multiple images makes the predictions of the AI tools far more accurate.
- Patient Monitoring Data: Remote patient monitoring devices and wearables collect continuous data on vital signs, activity levels, and other health metrics. This data can be utilized for real-time patient management and early intervention. Of course, we, as physicians, do not want to see every one of the 100k + heartbeats a human generates per day. We need to see what aberrations are. We need to see patterns of interest. This is where AI analytics can do a tremendous job. Abnormalities can be identified and flagged instantly.
- Genomic and Molecular Data: Genetic and molecular data are crucial in personalized medicine. AI can analyze genetic information to predict disease risks and tailor treatment plans. Public genomic databases like GenBank also provide valuable resources. Genetic data has major privacy considerations, as it can never be truly anonymized. However, when aggregated individuals blend into averages, population-based genetic changes can be found that might signal disease or risk of disease. There is tremendous work being done in Iceland right now, where over 75% of its citizens have added their genome into a massive database that can identify variations that might be linked to serious illness risk in a relatively homogeneous population.
- Natural Language Processing (NLP) Corpora: NLP tools require access to clinical notes, medical literature, and other text data for tasks like information extraction, sentiment analysis, and summarization. Our narratives in medicine are where much of the intuition about illness and wellness lies. AI tools, when consuming this, can begin to think like we do and find inferences that stand out, which may point to risk for individuals or populations.
- Population Health Data: Population-level data can help identify trends, outbreaks, and areas needing intervention. Public health agencies and research institutions often maintain such data. Usually, this data is kept separate due to issues of interoperability and even legislative barriers. There are great examples of advances that happen when this barrier is removed, though, as with the outputs of companies/health systems like Athena Health, where population and environmental data overlaying each other find outbreaks of Zika virus in a single neighbourhood of Miami or trends in pregnancy risk in women taking enalapril. Mapping illness information and environmental data will be even more important as the effects of things like climate change take their toll.
credit” @gapingvoid
While there is a significant amount of data available in healthcare, several challenges and considerations must be addressed to liberate it and make it fully useful:
- Data Privacy and Security: Healthcare data is sensitive, and patient privacy is a top concern. Compliance with data protection regulations like PIPEDA and PHIPA is essential.
- Data Quality and Standardization: Data quality and standardization are critical for AI model accuracy. Inconsistent or poorly documented data can lead to unreliable AI outcomes.
- Data Interoperability: Healthcare data often resides in siloed systems, making accessing and integrating data from different sources challenging. This remains the holy grail of health data repositories.
- Data Imbalance: Some datasets may be imbalanced, meaning they contain more examples of one class or condition than others. This can affect the performance of AI models, particularly in rare disease prediction and avoidance of very real flaws in algorithms.
- Data Bias: Biases in healthcare data, such as racial or gender biases, can lead to biased AI predictions and reinforce disparities in healthcare outcomes.
Efforts are underway to address these challenges and make healthcare data more accessible and usable for AI applications. These include data-sharing initiatives, data anonymization techniques, the development of data standards, and ongoing research to reduce biases in AI algorithms. Doctors need to be part of this analysis because, in the end, we are accountable for the decisions we make and the advice we give because of it. We must make space for this new thinking.
So, while a wealth of healthcare data is available, there are challenges related to privacy, quality, and bias that must be carefully managed to ensure the effectiveness and fairness of AI tools in primary care and healthcare. Collaborative efforts from healthcare institutions, data scientists, and policymakers are essential to harness the full potential of AI while addressing these concerns.
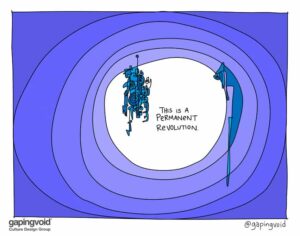
8 Replies to “Unraveling the Potential of AI in the Medical Practice How do we train the beast? An endless feed on data.”
Great explanation, thanks!
AI Ethics is one of my pursuits along with advocacy in mental health, identity, healthcare community engagement transitioning and cybersecurity.
Here again, it is vital to ensure Patient Partners (patient/user/consumer) are at the table.
AI Agents will play a key role I believe.
👍
Lyn I really appreciate you reading this article and for taking the time to comment.
Feel free to add in any ideas you have! The ethics behind AI in medicine are a thorny topic, from consent for data use to alerting patients of risk when they may well not want to know!!
We need more in this space!
One of my favorite articles you’ve written!
Thanks so much for saying so Charles.\
Kept the best for last! 🙂
Amazing content. I can appreciate the focus on finding where the data lies and making it available for AI models
I am certain! It is hard to make these data sets available for AI training in medicine. So many reasons why we “cannot” and so few incentives to show us we “can”.
Thanks for reading, Al!!
Great article against Darren – the value we can unlock makes wrestling AI worthwhile
This is so true Julia.
You can do this with BlueXBlue!!
Thanks for reading through!
D