Unravelling the Potential of AI in the Medical Practice: Data, Accountability, Bias and Regulation
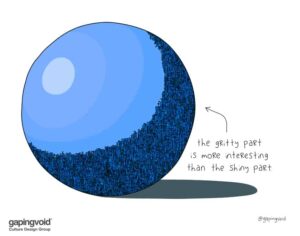
In my last three posts, I looked at AI in medical practice, unravelling some of the mysteries of what it is and is not and diving deep into trust issues. Many physicians are moving ahead with tools for their practices, primarily large language model-based, and if using a well-tested product, they are finding it relatively easy to implement. Others are struggling with the technology and changing workflows in figuring out how to use the tools efficiently. In the two posts that follow this, I will look at practical applications themselves, examining how we can move ahead more quickly, including what a future with AI in practice might look like. But before that, let’s delve into the challenges associated with integrating AI into the medical industry, mainly focusing on data quality, bias, accountability and complexities surrounding AI recommendations.
Data Quality and Reliability:
First, we must remind ourselves that AI tools are not built from thin air. They begin with information from existing sources that is read, massaged, interpreted, and put into algorithms that bring insights to light. Often, algorithms use normalized data sets aggregated in institutions like hospitals. There is very little liberation of data from the community in that each of our EMR systems is a very small, closed loop. Data is local. It is rarely shared. We cannot train the AI engine on our specific practices very easily.
One of the paramount challenges in an AI-driven medical world is ensuring the quality and reliability of the data that feeds into AI systems. Inaccurate or incomplete data can lead to erroneous conclusions and recommendations, potentially putting patients at risk. Therefore, healthcare organizations must invest in data governance and quality assurance processes to maintain high data standards. This is difficult work. Governance conversations often take years. Standards are often far from standardized. And it seems that many institutions want to work alone in their silos, stating laws that prevent data sharing due to privacy concerns.
Moreover, medical data is often scattered across various sources, including electronic medical records, wearable devices, and patient-reported information. Integrating and normalizing this diverse data is a complex task that requires meticulous data management. Many institutions, especially community practices, lack the know-how and manpower to make this happen. Hiring professionals and data management firms is very expensive and beyond the reach of most practitioners. Because of this, we often rely on AI engines from major technology players and have little knowledge about how their products are trained. This may lead to major issues if our patient population is “not average” or if our practices are special, and most truly are!!
Transparency and Interpretability:
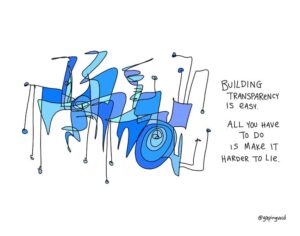
AI algorithms, especially deep learning models, can be highly complex and difficult to interpret. Ensuring transparency in AI-driven medical decisions is essential for gaining the trust of healthcare professionals and patients. When AI systems provide recommendations or diagnoses, explaining how they arrived at those conclusions is crucial.
Currently, AI’s “black box” nature is a significant challenge. We cannot often fully understand the inner workings of AI algorithms, making it difficult to determine how they reached a particular medical decision. Addressing this challenge involves developing model interpretability methods, allowing professionals to see the rationale behind AI recommendations. This is particularly important when AI is involved in high-stakes decisions, such as specialized treatment plans or high-risk environments.
Accountability and Oversight:
Accountability becomes critical as AI plays an increasingly central role in healthcare decision-making. While doctors are held accountable for their actions and decisions, ensuring similar accountability for AI systems is challenging. Determining who might be responsible when an AI system makes an incorrect or harmful recommendation is not straightforward.
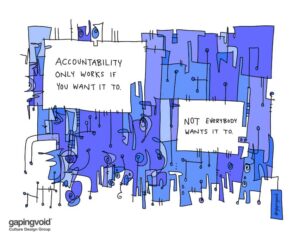
The rapid advancement of AI in healthcare has outpaced the development of comprehensive regulatory and legal frameworks. To address this, governments, regulatory bodies and healthcare organizations must establish clear guidelines and standards for new AI accountability. This includes defining the roles and responsibilities of healthcare professionals and AI developers and establishing protocols for auditing AI systems. Are our governing bodies ready for this? Can they keep up with the pace of change?? Time will tell. The main goal is to ensure that AI is used as a supportive tool, augmenting the expertise of medical professionals rather than replacing it. Clearly, the ultimate responsibility for patient outcomes remains with human caregivers – our doctors and nurses. This can be an unacceptable burden.
Ethical and Bias Considerations:
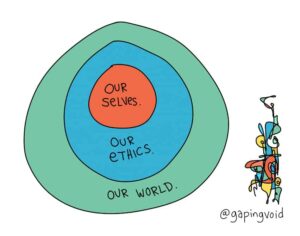
AI algorithms can inadvertently perpetuate biases in the data they are trained on. In healthcare, this can result in disparities in diagnoses and treatment recommendations. To address this challenge, it’s crucial to implement bias detection and mitigation techniques and diverse and representative training datasets. We have no tolerance for a system that is racist, for instance or perpetuates inequities of any kind.
Additionally, ethical questions arise when AI is used to make difficult decisions in vague situations, such as resource allocation during a pandemic or end-of-life care choices. Balancing the efficiency and impartiality of AI with ethical considerations requires careful deliberation and may need the involvement of ethicists and policymakers. Our tools will need to give us a variety of choices, with probabilities that they are correct, so that we can better make decisions, Often patient choice will be the final determinant.
While the potential benefits of AI in the medical industry are undeniable, the path to realizing these benefits is riddled with challenges. Addressing data quality, transparency, accountability, ethics, and regulation issues is essential to harness AI’s full potential while safeguarding patients’ well-being and maintaining healthcare professionals’ trust. The journey ahead requires a collaborative effort from healthcare providers, technologists, government policymakers, and ethicists to navigate these challenges effectively. Until then doctors are left with the principle of “caveat emptor”, or “buyer beware” when implementing tools in practice. We need to take the time to ask the right questions about the products we use, and this job is not easy, especially when neither the questions nor answers are clear.
Stay tuned for Part 5: What AI Tools exist for Primary Care and Specialist Clinics?
2 Replies to “Unravelling the Potential of AI in the Medical Practice: Data, Accountability, Bias and Regulation”
Love this! As someone who’s spent the last 4 years building AI for hospital coding workflows, the data integration piece is the most critical AND challenging step.
A few comments on this:
– on the facility/hospital side, data integration is highly dependent on IT/interfacing teams, who are usually under resourced and over capacity with projects. If a data integration/extraction is too complex or time consuming, it can take up to a year to complete the interface (with all of the delays). This slows down the time to deployment and scaling of AI systems
– on the topic of incomplete and inaccurate data, this is critical to mitigate. Errors in coding or documentation distort our understanding of what happened to the patient and how they were treated. There are AI systems (like the one I’ve been building) that “audit” or “spell check” the documentation and coding for accuracy. So I think this is a good starting point for AI
– community based EHRs can really go a long way to accelerating speed to data integration for AI solutions. Standard and modern APIs are going to be key differentiators for these EHRs versus legacy interfacing (like HL7 and FHIR).
We’re solving a few of these challenges – simplifying the data extraction workflow for larger facility based EHRs, auditing coded/clinical data with AI for “spell checking”, and working with community based EHRs on modern data APIs that will help differentiate them.
Please reach out if you’re curious!
This is so true Nick!! ! I have always wondered what a product like the one you built could do in the community EMR space, especially about cleaning up the data we generate, coding it, and making it available for use in everything from quality improvement to system-level planning!! Data quality could be improved so much by something like Semantic. Of course, there is no business case for this as no one wants to or can pay, and currently, there is no financial incentive (or even non-financial!!) for docs to deliver data anywhere . So, we are at a standstill.
I am interested in how you see the modern data API as a sub-in for data standards like FHIR. I have always thought of an API as a point-to-point product that requires building or customizing every single time being harder than data standards that allow movement through a more open API or comms channel. Teach me please!
What can we say about IT teams and the time required to do integration work? You hit the nail on the head. It is one thing in the hospital (taking forever as you noted) and another thing completely for the community, where there is no IT team at all!! Is there a role for corporate Canada here?? Or for a federal or provincial agency to step in? I just don’t know how we move ahead in primary care or community practice, where 80% of all the care in Canada is provided, if there is no higher level of coordinated effort.
Thanks a lot Nick, for adding to this important convo! And yeah, I’d love to chat about this more!! Consider you reached out to!! 🙂