Unravelling the Potential of AI in the Medical Practice: Is AI just a fad??
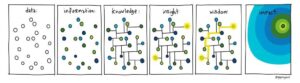
Like anything new, some doctors have told me that AI in medicine is a fad. It will never be able to do what a physician does. To a degree, for the next few years, they are correct. But AI is not just a fad; it represents a significant and enduring technological advancement with far-reaching implications across various industries, including medicine. I want to think about how “sticky” AI is, in the context of the ability of these tools to become integrated and indispensable components of healthcare practices over time. Here are some areas where we see very real progress in AI in medicine:
- AI tools have already shown utility: AI tools in medicine have demonstrated their utility in various applications such as disease diagnosis, treatment recommendation, drug discovery, and medical imaging analysis. They have shown the potential to improve the accuracy and efficiency of healthcare processes. Many of these processes are business-related or acute care-oriented, where numbers are key, and processes are reproducible. But generative AI can change that in that it can use much softer information to make predictions and do work for us. And it can learn.
- Clinical integration: Many healthcare institutions and providers are actively integrating AI tools into their clinical workflows. These tools can assist healthcare professionals in making more informed decisions, reducing errors, and providing better patient care. We are all familiar with large language models like Chat-GPT. These are now being used alongside EMRs to listen to a clinical encounter, transcribe it and then convert it into a complete clinical note. Decision support will quickly follow this. AI is used to predict sepsis in infants in the NICU, to alert us of a compromise in the OR and even to help map infectious disease to weather patterns and postal codes.
- Research and development: AI is playing a significant role in accelerating medical research and drug development. Machine learning algorithms can analyze vast datasets to identify potential drug candidates, find rare diseases, and predict disease outbreaks. For instance, it’s now clear that a Canadian AI company, Blue Dot, predicted the COVID-19 pandemic before it was on anyone’s radar in North America.
credit: @gapingvoid
- Patient-centric care: AI tools are helping to shift healthcare towards a more patient-centric model by enabling personalized treatment plans comparing personal data to norms and finding the best path forward. Patients can benefit from tailored interventions and better management of chronic conditions. Tools can monitor, track, alert, and recall. They can bring knowledge to an information gap between doctors and patients.
- Cost-efficiency: AI tools have the potential to reduce healthcare costs by optimizing resource allocation, streamlining administrative tasks, and preventing medical errors. The economic benefits can incentivize the continued adoption of AI in healthcare. Even though this works best on the institutional level, it will not be long until smaller analytics can be used in community practice. We will better understand our costs in doing business. We will predict where to spend better. And we will be able to become more efficient doing the same work in less time or doing better work in the same time.
- Regulatory frameworks: As AI tools in medicine become more prevalent, regulatory bodies are developing frameworks to ensure their safety and effectiveness. This oversight may seem too little too late, but it is happening. Regulatory oversight provides a level of assurance that can promote stickiness in the field. Good regulation can go a long way to protecting citizens and healthcare professionals from mistakes that could come from wanton AI use. It helps us build trust.
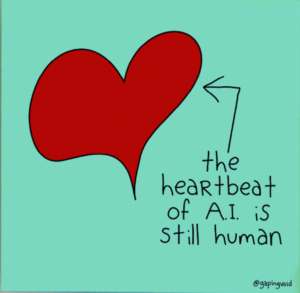
- New AI model development: The field of AI in medicine continues to evolve with ongoing research and innovation. New AI models and applications are regularly being developed and tested, further solidifying their role in healthcare. In Canada, we are lucky to have three major global centres of AI research: The Vector Institute in Toronto, MUNI in Montreal, and AMII In Edmonton. These are world-class. And each of them is working on medical applications.
- Data Availability: The increasing availability of healthcare data, not just its collection but its liberation for use, combined with advancements in AI, creates opportunities for continuous improvement in diagnostic accuracy and treatment recommendations. This data-driven approach enhances the stickiness of AI tools. Even our unstructured notes can now be consumed and understood. Access to coded data becomes less and less of a limitation.
While AI in medicine is not without challenges, it is not just a passing trend in the healthcare industry. The proven benefits, ongoing research, and integration efforts suggest that AI tools will continue to play a vital and enduring role in improving healthcare outcomes. We must pay attention. We will need to figure out not if AI will be used in our practices, but how
Stay tuned for Part 2: Trust, Mistrust and Distrust.
Posting next week!
Subscribe to have it sent right to you!
9 Replies to “Unravelling the Potential of AI in the Medical Practice: Is AI just a fad??”
Have you adopted AI in your practice lately?
HI Darren, thanks for bringing the big AI elephant in the room into some light, and giving each of us blind medical folk (and the public at large) a feel of the different parts. I would tend to agree with you that AI has enduring power and utility. As you’ve noted, it tends to work well in those situations of where data, especially large and complex sets/presentations, benefits from distillation. AI can be fine tuned to ‘learn’ from increasing the data quantity and fuzziness, much like physician experience. Much hope and excitement of a more efficient, accurate and better future awaits us.
However, there are cautions to be noted. First off, while superior with hard data analysis, querying, collation and prediction, the human, social, and biologic presentations are often not hard, but rather soft, incomplete and quite fuzzy. The underlying power of AI is driven by 2 things, simplistically speaking. There is/are the data set(s), and there is/are the algorithm(s) that work the data. Any AI is only going to be as good as these underlying components. I recall the unverified but plausible story of Apple’s introduction of FaceID to their iPhones. Broadly tested, richly produced, and keenly marketed. Worked fairly well in our Western markets, but Asian users seem to have many stories of inadvertent FaceID unlocking by siblings or any number of people that ‘look alike’ (😉speaking as a Chinese guy). The facial data sets and algorithms had to be enhanced and tweaked for further/different characteristics.
Second, as physicians, we often refer to a sixth or Spidey sense, or intuition, that experience affords us. That may be drawing from a disconnected experience, purposely breaking a ‘rule’, or having a heightened/weighted sense of concern. Our internal clinical data sets are sometimes seemingly irrelevant, distant, diffuse; until they’re not. And our algorithms are dynamic.
Third, much of our clinical value is driven by moral and ethical subroutines; something we have not discussed enough about how to integrate that into AI functionality.
And perhaps my final caution of the night, is to note that may instances of AI output is so good, it’s hard to spot their mistake, deficiency, or misdirection. Any of us who have been preceptors to students and residents know that a deficient learner while stressful in time expenditure, is in fact, fairly easy to manage in terms to their decision capacity; don’t trust most of it. A competent learner is more efficient, but more stressful in that I am uncertain about the dependability and correctness of their decisions. Along side with this growing tide of AI capacity, we need evaluative methodologies to ensure quality, accuracy, relevance and dependability. And we need this in the context of the presentations at hand.
And with this, I look forward to your next week’s musings. Cheers.
Norm, such a thoughtful and deep response to this posting… thank you so much!!
Thank you, as well for raising the cautions that you did. These are real and some of them are covered in the articles I will be posting over the next five weeks. We have to get these right to build confidence that more advanced AI tools will serve us, not put us at risk.
I get excited about the idea that data generated in our own offices could be used to train more local instances of AI algorithms, or be moved into larger data sets to contribute to even bigger training datat sets. This could super charge our work in population health, accountability and especially system planning.
Your examples are excellent ones. It’s clear you have walked the walk here! And we can all learn from these as we examine how AI is approached by us as physicians.
Keep your eyes open for parts 2 – 5 Norm!!
Cheers!
I love this! Clinical medicine is so nuanced and complex, and half art as it is science. So, I don’t think AI will ever replace physicians but rather enhance them. AI is still in its early days in healthcare, but thinking about the AI-clinician relationship – what that looks like and how that should evolve – is super important for us to do now!
Thanks a lot for reading and commenting here Nick!!
AI has to enhance the work of physicians. There is no other way. The very earliest iterations of tools for practice will be scribes and ambient sensing technology that help us with charting. This functions fairly well now and will only get better. And having that good start will build trust in tools that follow. All worthy relationships are built on trust.. and AI tools have to EARN our trust. This is not offered over by physicians easily. How do they gain trust? By being TRUSTWORTHY. This will involve transparency, consistency, integrity and reproduceability so that we can have confidence in the decision we make using it.
I would be excited to hear how you think this relationship should or could evolve Nick!
Very interesting! It’s eye opening to hear how AI is already in use in the medical industry. Although, I’m a bit sceptical of AI being able to predict pandemics as they are so infrequent. Like weather, disease spread is chaotic, meaning that small day-to-day events can have a huge impact on the overall disease’s spread. That being said, AI has already proven itself useful in many applications. I’m most excited for it to be combined with tele-health to drastically reduce the burden on the healthcare system and improve the patient experience. Looking forward to the next post!
Great insights Daniel!! Thanks for throwing your thoughts into this conversation. I know you have thought deeply about the applications of AI in the real world. In fact, I am proud to say that you taught me most of what I first learned in understanding neural networks!! It is interesting the perspective of AI being used in virtual care. We have a long way to go beyond basic chatbots available now, but I am confident that advancing LLMs and even emotion capable virtual humans could take on a lot of the simple advice doctors and nurses give now. When we can trust the content fully of course!!
I hope you keep reading and commenting, making me smarter!
D
I was forwarded this excellent article by a cancer researcher. I’m currently engaged in a review process with the CMA that is looking at supporting AI innovations. I have found though that often the patient perspective is being left our of these discussions. I wrote an article that was well received though not peer reviewed on the patient perspective in lung cancer. https://www.ilcn.org/artificial-intelligence-in-healthcare-a-patient-perspective/
I would note that the NEJM in their new AI journal has seen fit to include a regular Patient Perspective column.
Angus
Angus… thanks so very much for reading this blog post and for offering your perspective. YES, as in almost every aspect of healthcare the patient perspective is not considered first line as it should be. When I was at OntarioMD, very much aware of this problem, I created a “Patient Leader Program” for health technology projects in Ontario. This program still exists under the OMD Peer Leader banner. The whole idea was to have a tech friendly patient advisory group that could be used by big companies and small as they drive out their new innovation and product lines. Involvement from the very first concept ensures that we have the right products being built in real time, not having to jerry rig after the fact when most of us think to ask the patient perspective. I was also very aware that we always had bias in our patient panels. Those who could take part were not usually the most challenged in our health care system who struggled with three jobs and endless work just to keep food on the table. You think they have time to attend our all day meetings?? Even though we paid them well for their time the answer was no. They had commitments.
I will eagerly read the patients in AI article you sent. Thank you for doing so.
I also found your blog called Journey. It is a fantastic read. I appreciate all you do to make us and our systems better. Please read more as I publish it!!
And be well.